Author ORCID Identifier
0000-0002-0153-6144
Document Type
Article
Disciplines
Computer Sciences, Bioinformatics
Abstract
Background: Creating models to differentiate self-reported mental workload perceptions is challenging and requires machine learning to identify features from EEG signals. EEG band ratios quantify human activity, but limited research on mental workload assessment exists. This study evaluates the use of theta-to-alpha and alpha-to-theta EEG band ratio features to distinguish human self-reported perceptions of mental workload. Methods: In this study, EEG data from 48 participants were analyzed while engaged in resting and task-intensive activities. Multiple mental workload indices were developed using different EEG channel clusters and band ratios. ANOVA’s F-score and PowerSHAP were used to extract the statistical features. At the same time, models were built and tested using techniques such as Logistic Regression, Gradient Boosting, and Random Forest. These models were then explained using Shapley Additive Explanations. Results: Based on the results, using PowerSHAP to select features led to improved model performance, exhibiting an accuracy exceeding 90% across three mental workload indexes. In contrast, statistical techniques for model building indicated poorer results across all mental workload indexes. Moreover, using Shapley values to evaluate feature contributions to the model output, it was noted that features rated low in importance by both ANOVA F-score and PowerSHAP measures played the most substantial role in determining the model output. Conclusions: Using models with Shapley values can reduce data complexity and improve the training of better discriminative models for perceived human mental workload. However, the outcomes can sometimes be unclear due to variations in the significance of features during the selection process and their actual impact on the model output.
DOI
https://doi.org/10.3390/biomedinformatics4010048
Recommended Citation
Raufi, Bujar and Longo, Luca, "Comparing ANOVA and PowerShap Feature Selection Methods via Shapley Additive Explanations of Models of Mental Workload Built with the Theta and Alpha EEG Band Ratios" (2024). Articles. 230.
https://arrow.tudublin.ie/scschcomart/230
Funder
No funding was received for this work.
Creative Commons License
This work is licensed under a Creative Commons Attribution 4.0 International License.
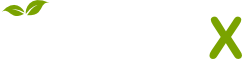
- Citations
- Citation Indexes: 2
- Usage
- Downloads: 29
- Abstract Views: 6
- Captures
- Readers: 8
- Mentions
- Blog Mentions: 1
- News Mentions: 1
Publication Details
https://www.mdpi.com/2673-7426/4/1/48
Raufi, B.; Longo, L. Comparing ANOVA and PowerShap Feature Selection Methods via Shapley Additive Explanations of Models of Mental Workload Built with the Theta and Alpha EEG Band Ratios. BioMedInformatics 2024, 4, 853-876.
https://doi.org/10.3390/biomedinformatics4010048