Author ORCID Identifier
https://orcid.org/ 0000-0001-6462-3248
Document Type
Article
Rights
Available under a Creative Commons Attribution Non-Commercial Share Alike 4.0 International Licence
Disciplines
Computer Sciences
Abstract
In this paper, we compare and assess the efficacy of a number of time-series instance feature representations for anomaly detection. To assess whether there are statistically significant differences between different feature representations for anomaly detection in a time series, we calculate and compare confidence intervals on the average performance of different feature sets across a number of different model types and cross-domain time-series datasets. Our results indicate that the catch22 time-series feature set augmented with features based on rolling mean and variance performs best on average, and that the difference in performance between this feature set and the next best feature set is statistically significant. Furthermore, our analysis of the features used by the most successful model indicates that features related to mean and variance are the most informative for anomaly detection. We also find that features based on model forecast errors are useful for anomaly detection for some but not all datasets.
DOI
https://doi.org/10.3390/iot3010008
Recommended Citation
Agrahari, Rahul, Matthew Nicholson, Clare Conran, Haytham Assem, and John D. Kelleher. 2022. "Assessing Feature Representations for Instance-Based Cross-Domain Anomaly Detection in Cloud Services Univariate Time Series Data" IoT 3, no. 1: 123-144. DOI: 10.3390/iot3010008
Funder
Science Foundation Ireland
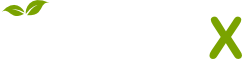
- Citations
- Citation Indexes: 6
- Usage
- Downloads: 161
- Abstract Views: 33
- Captures
- Readers: 7
- Mentions
- Blog Mentions: 1
Publication Details
IoT